An Attribution Model Comparison: Shapley and Markov vs. Corvidae
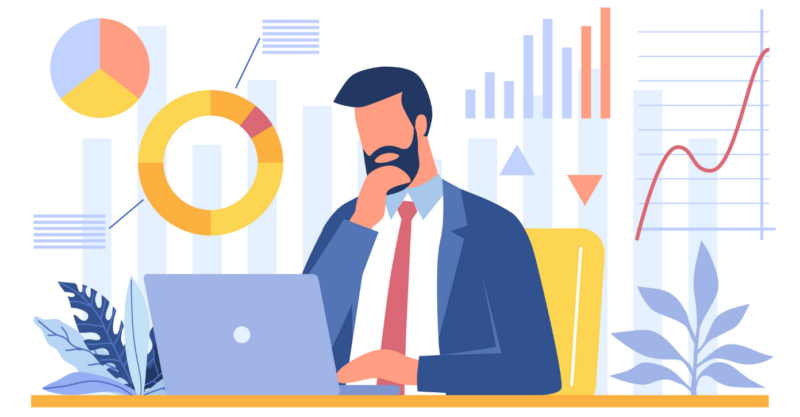
Attribution models are central to delivering highly effective marketing that contributes to revenue and ROI inside any business.
And there are a plethora of them out there from simple rules-based approach to data driven models like Shapley and Markov and beyond.
In this blog, we look at the benefits and limitation of these types of approaches whilst comparing them to the innovative approach taken by our own Corvidae platform, which leverages cutting-edge technology like Machine Learning and AI to really change the game in attribution model terms.
But first, let’s start with some quick context that sets us up for a wider analysis.
Simple attribution models simply don’t cut it
Limitations in simple rules-based attribution models – like Last-Click (which is still the default model in Google 360), First-Click, Linear and Time Decay models – mean they lack the sophistication needed to capture the complexity of increasingly complex buying journeys. Journeys that are happening on and offline, across a raft of devices and are being influenced by a plethora of media from in-store digital promotions to re-targeting ads.
They also, by definition, have inherent biases to specific parts of the funnel that have driven some marketers to explore data driven-models in the search for more effective attribution.
These predictive, data-driven models are much better attuned to handling the nuances that exist in complex customer journeys. And have a focus on aggregating data and modelling how touchpoints actually influence conversion as part of the wider customer journey.
Two of the more common ones are Shapley and Markov – and we take a quick look at each in the next section.
Introduction to Shapley – what we’ve learned
Shapley is a predictive attribution approach based around game theory.
Essentially the concept being that several “actors” can work together co-operatively towards a common goal or payoff – with a focus on effectively valuing the marginal contribution each exerts in the process.
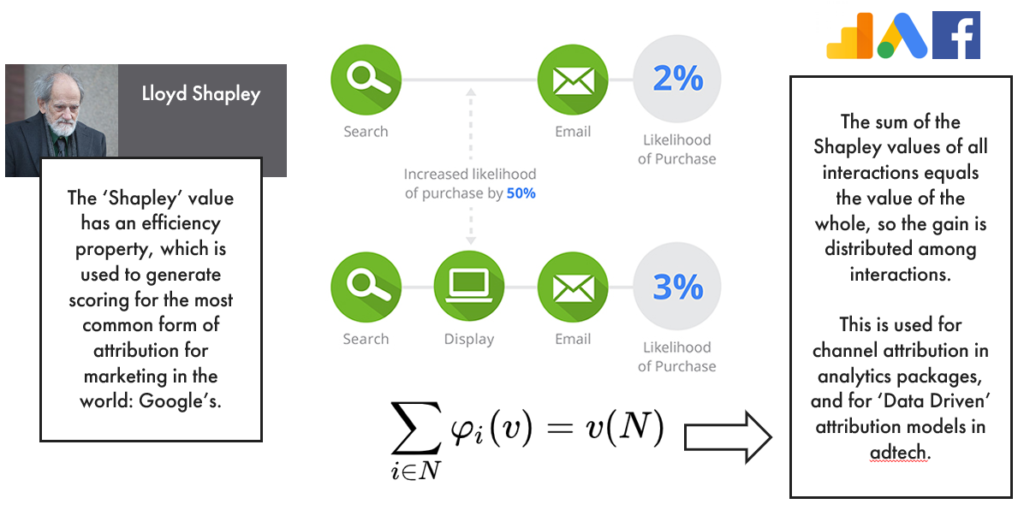
In marketing attribution terms, channels and campaigns are the actors that work collaboratively to influence marketing conversion.
A very broad-brush explanation of a typical Shapley approach is to find an average incremental value for a particular channel touchpoint by looking at all conversion paths that exist, as well as their conversion path rates and their mix of channels. These are then compared to create an average incremental value for each channel for each conversion path. This then feeds back into channel and campaign analytics.
Benefits of the Shapley attribution model
- It can improve the quality of insight into the performance of marketing channels
- It takes a fairly equitable approach to applying contribution impact across channels
- If channels make zero impact this is reflected in contribution scoring
Limitations of the Shapley attribution model
- The number of possible sequences and interactions is potentially huge
- The huge level of data required for modelling makes it computationally expensive with a need to remodel to provide more granular outcomes than just channel level attribution
- Its’ very nature means that channels that appear infrequently in the journey can be undervalued
- It is not possible to model small paid campaigns with this type of approach
Introduction to Markov – what we’ve learned
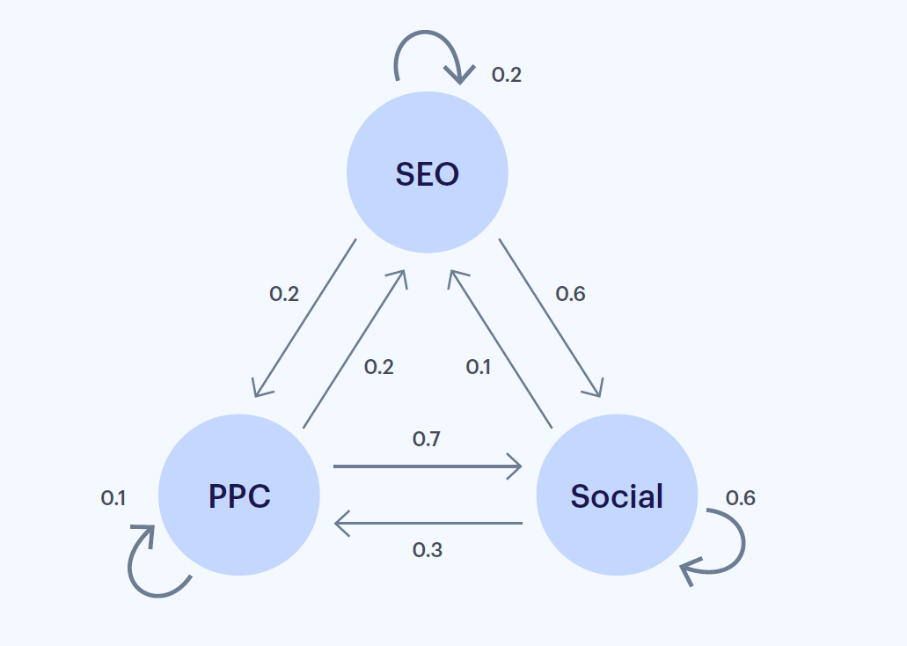
A second predictive attribution approach that we also have experience of using is Markov Chain.
Markov Chain is an example of a sophisticated, probabilistic modelling approach that looks at individual events in the customer journey and identify patterns of user behaviour to build journey profiles.
By evaluating the probability to move from one state to another in the journey.
For example, if a website visitor is identified as coming in on Organic Search, the Markov Chain would then understand and look at the other channels the visitor could have come in on. And the likelihood they would come back from Organic. It would also look to see if they would come from Paid or if they would never come back or if they would transact.
And use the resulting values to effectively attribute the impact of specific media.
Again, we have used Markov in the past. So, what are the benefits of this approach?
- One benefit over Shapley is that is takes account of the random nature of the funnel – it’s not purely linear
- It is possible to revisit the effectiveness of the model and its accuracy by comparing what you predicted and what you get
Benefits of the Markov attribution model
- Where you have long journeys with many channels there is a danger that channels are over-weighted
Limitations of the Markov attribution model
And like Shapley it also falls prey to the issue around large data sets and the sheer cost and time associated with constant re-modelling of data.
So, Shapley and Markov both have pros and cons. But what if the focus on choice of attribution model is masking other considerations that are just as – if not more – important? Like the quality of data that you are feeding into them?
What if attribution models are only part of the story?
We know that data quality is a key concern for many marketers. In fact, our recent survey of Performance Marketers in the retail sector found that over 60% of retail marketers think data to support cross-channel decision-making is broken.
In our early days of working with clients we used Shapley and Markov for attribution but what we found was that they generated fantastically different results depending on the data used. And, crucially, whether or not you rebuilt your data BEFORE you applied the attribution.
Take the example below, which is a real-world example for work done with our client leading UK retailer Tesco. In this case we compared the relative attribution performance of Google Analytics 360 (which uses the Shapley model for attribution) with the approach that Corvidae takes which includes rebuilding the core data before performing more effective attribution.
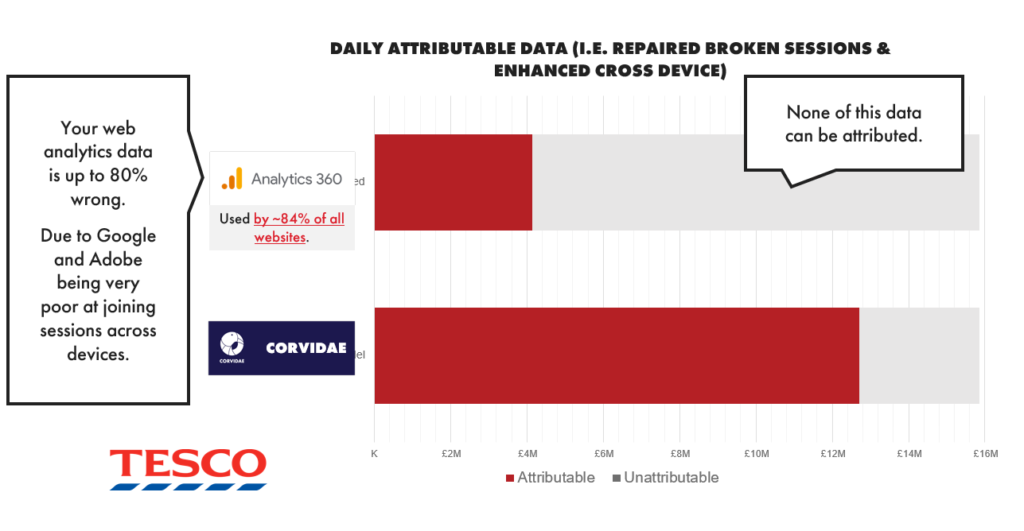
The results are fairly self-explanatory, with up to 80% of the data incorrectly categorised in the Analytics 360 approach.
So what is different about the approach that Corvidae is taking to achieve this?
Corvidae rebuilds your data from the ground up
The issue above is caused by the well-documented poor job that cookies do of effectively tracking individual users across complex, cross-device journeys.
And this was one of the early learnings in our journey as a business to a much better way of performing attribution on behalf of customers. And the genesis of the idea behind Corvidae. A strong realisation that in a lot of ways the choice and relative sophistication or effectiveness of your attribution model is a bit of a moot point – if you are essentially putting data from lots of broken journeys into it.
So, the first priority is to rebuild your data – before you apply attribution. And that is what Corvidae does. It uses innovative session stitching technology to rebuild your data from the ground up. Effectively at this point we are replacing the cookie. And what Corvidae is able to do at a granular level is create a map of every interaction that an individual has – in a privacy respected environment. Then put data alongside it and identify markers, like time series and location, and “stitch” ads and media into the conversion journey.
LSTM provides more powerful attribution model than either Shapley or Markov
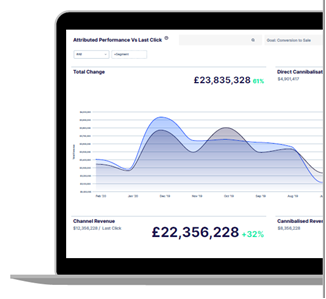
At the centre of our approach to attribution – effectively our attribution “model” that replaces Shapley or Markov – is LSTM (Long-Short Term Memory). LSTM is a type of recurrent neural network capable of learning order dependence in sequence prediction problems.
We undertook a two-year project with Edinburgh University which looked at applying Machine Learning techniques (Random Forest in this case) and the principles of LTSM to create technology that applies the deep learning techniques to the issue of marketing attribution.
And it has a number of really significant benefits including:
- You are able to “teach” the model over time, because you are working with something that works like a brain and learns over time
- And as you teach it, it gets more accurate
- Because is has a legacy of learning, it is possible to get up to speed within 30 days because it has already had quite a lot of training on similar data sets
It also removes some of the data restrictions that have dogged Shapley and Markov because it is possible to work with smaller data sets that are much more accurate. Which also opens the door to campaign and creative level attribution analysis that simply isn’t an option in either of the other two models. Due to cost and resource issues.
So LTSM effectively is the attribution model inside Corvidae.
So why does this all matter?
At the start of this blog, we opened with a recognition that effective attribution in itself is about effectively measuring the contribution of each and every ad and campaign creative to establish the impact it is having. And ultimately its impact on overall business ROI.
Shapley and Markov might get you part of the way into improving your attribution view. However, as we have seen, without giving due consideration to rebuilding your data how valuable is that really?
And in a highly competitive market, where a really significant proportion of ad buying is auction-based and geared towards buying on Facebook and Google. Anything that helps you move up the funnel – because you can accurately attribution the impact of higher funnel activity – is gold dust. As that’s where you can acquire customers more cheaply.
It also keeps you away from a crowded lower funnel which is competitive for a number of reasons including:
- People are closer conversion to which means advertisers know that quickly after they try and influence them they can expect results. Which pushes prices for this type of activity up.
- It’s also competitive because most people don’t have effective attribution in place. And so, the data that they’re using, by default is data that’s closer to conversion. Because they’re not fixing the cookie problem. And are focusing all of their activity, either implicitly or explicitly on that moment before conversion. Simply because the data quality breaks down very quickly, as soon as you try and move up to earlier in the funnel.
Corvidae, data rebuilding and LSTM enable you to step away from that spiral and focus on finding higher value, lower cost opportunities further up the funnel to boost your effectiveness and marketing ROI.
In recent case studies for example, Corvidae has found media spend reallocation opportunities giving 20:1 ROAS in Google Ads, and 35:1 in Facebook. If you’d like to find out more about our innovative attribution technology, click the link below.
Discover Corvidae
For Attribution That Works.
Own your marketing data & simplify your tech stack.
Have you read?
I have worked in SEO for 12+ years and I’ve seen the landscape shift a dozen times over. But the rollout of generative search engines (GSEs) feels like the biggest...
As you will have likely seen, last week Google released the March 2024 Core Algorithm Update. With it, comes a host of changes aiming to improve the quality of ranking...
After a year of seemingly constant Google core updates and the increasingly widespread usage of AI, the SEO landscape is changing more quickly than ever. With this rapid pace of...