How Artificial Intelligence May Be The Key To Making Marketing Attribution Work
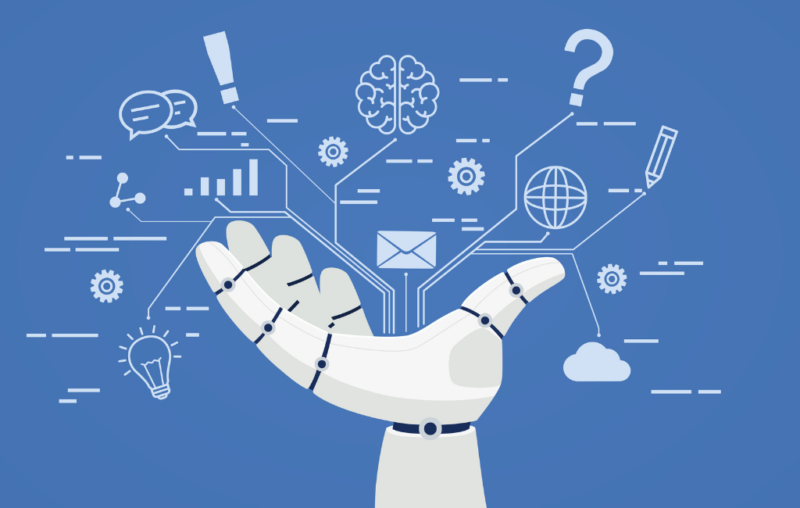
There are now hundreds of revenue attribution tools available to marketers. Some are from global corporations. Many more are from start-ups who want to grab a slice of a fast-growing software market that one analyst believes will be worth $3.6 billion by 2023.
Google offers GA360, Adobe has a similar product. These two companies dominate the market. HubSpot, Pardot, and even Amazon have also launched revenue attribution tools in the last few years, but they don’t tell you much about what’s going on outside of their own ecosystems. And it would be shocking indeed if Facebook reported that channels other than its own were delivering the best return on your advertising dollars.
So, here’s the problem. Most marketers don’t trust what the tools are telling them, in part because none of the tools is capable of helping them see the bigger picture by analysing the varying types of data that are fed into them from online and offline marketing campaigns. And because they don’t trust the data, they are forced down blind alleys, over-investing in expensive PPC campaigns, under-investing in medium- to long-term brand building, and failing to truly optimise marketing investments.
Let’s start with the data
Marketers face no shortage of data. In fact, they’re drowning in it and it’s a significant challenge to understand both the nature and accuracy of the data with which they’re presented.
Data can be classified as deterministic or probabilistic.
- Deterministic data is easy to understand and trust. If you send someone an email with a hyperlink embedded somewhere in the text and they click on the link, it’s almost certain that the intended recipient is the person that actioned the click. You can measure the percentage of people that click and, possibly, track them through to a purchase. Their direct behaviour and unique identifier combine to produce an accurate picture. Such data can come from other sources too: customers that log into your website, either via an app or browser, people that complete an online form, some types of in-store data collection, and other channels that meet the required criteria.
- Probabilistic data is more difficult to analyse and interpret because it’s all about making estimates. In order for estimates to be accurate, it’s important that there is sufficient data and that it comes from representative sources. In other words, care needs to be taken to ensure that the audience from which the data is sourced represents the customers you want to reach and that you have enough data to ensure that the resulting analysis is statistically robust. You don’t want to omit relevant groups, or over-represent others if the resulting data is to be useful in optimising marketing investments. Probabilistic analysis is the only viable way to determine the effectiveness of linear radio/TV, print advertising, outdoor campaigns, and some aspects of both social media and in store advertising.
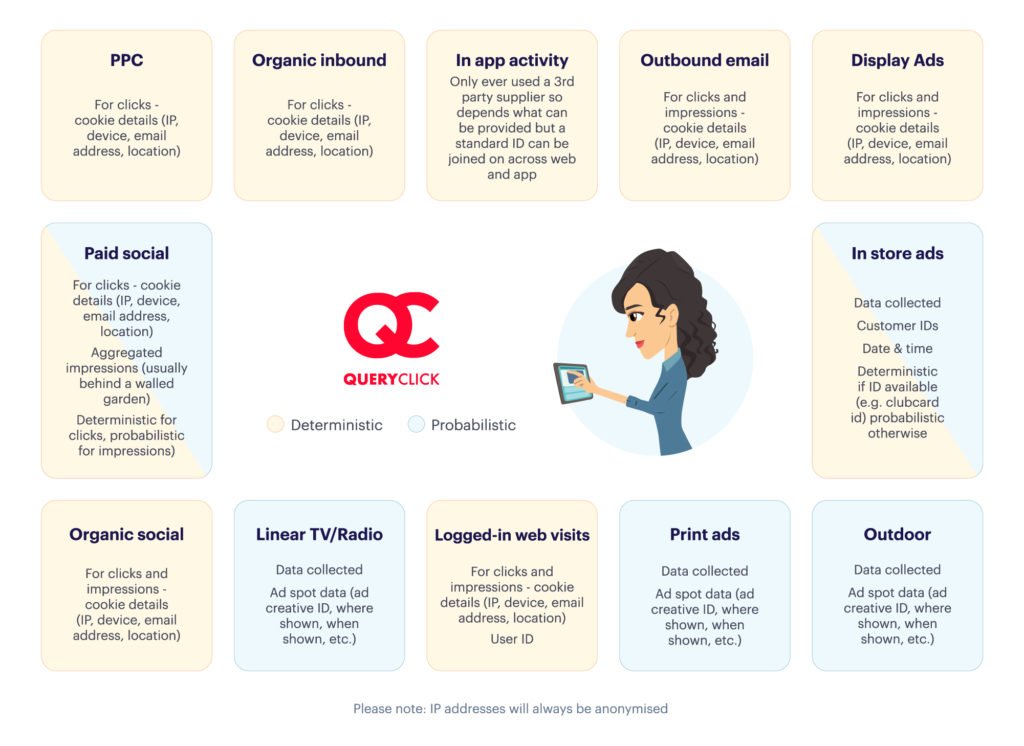
Poor quality data could be impacting your attribution. Find out why…
What AI can do to help
Artificial intelligence, or AI, is a generic term used to describe computing systems that attempt to emulates how our brains work. While we think of today’s AI systems as being highly sophisticated, they are still relatively crude. They exhibit a tiny fraction of the overall capabilities of our brains and consume many times more power. The human brain runs at about 20 Watts; computers consume thousands of watts to perform much less work.
Nevertheless, AI, specifically in the form of machine learning (a subset of AI), is much better at some tasks than we are. This includes taking vast volumes of data, analysing them, and presenting the results in ways that help us see patterns we might otherwise miss. If your maths is good, this blog post by IBM is a great introduction to AI terminology and principles. There’s more of a plain English guide to machine learning and algorithms by data analytics firm, SAS, here.
Machine learning (ML) can play three important roles with respect to marketing attribution data: The first is to clean up ‘dirty’ data that you may have assumed to be deterministic but isn’t. The second is to analyse probabilistic data. The third, and most exciting, is to marry deterministic and probabilistic data together to reveal the behaviour of consumers throughout their buying journey.
- Cleaning up ‘dirty’ data that you may have assumed to be deterministic but isn’t
- Analysing probabilistic data.
- Marrying up deterministic and probabilistic data to reveal the behaviour of consumers throughout their buying journey.
In a world where most marketing attribution tools can only really provide information about what’s happening at the level of an individual browser, this final point is crucial. Browsers aren’t buyers, people are. It’s important to understand the limitations of tracking browser cookies and pixels. Without such an understanding, you’ll waste a significant proportion of your marketing budget by making poor decisions based on poor data.
Learn more about how Machine Learning can accelerate your digital marketing strategy
Random Forest – a proven algorithm for cleaning up your act
Google Analytics, and to a lesser extent Adobe Analytics, are the tools of choice for most digital marketers. Google’s GA360 toolset is often the obvious choice, not least because so much marketing effort is directed towards generating website visits through Google’s search engine. There is a natural assumption that the data produced GA360 must be reasonably accurate.
But, as a famous line from the US TV show ‘The Odd Couple’ proclaimed:
“Never ASSUME, because when you ASSUME, you make an ASS of U and ME”
Independent research has repeatedly shown that the raw data extracted from Google Analytics tools is often highly inaccurate. Digital marketing agency, QueryClick, has found the raw data from website sessions and display advertising used for marketing attribution is often up to 80% inaccurate, which goes some way to explaining why marketers struggle to find causative links between marketing actions and attribution data. Many factors contribute to this inaccuracy. The data from one browser session may be accurate, but it doesn’t tell you who is using the browser. Then there’s the fact that buyer journeys often involve multiple online sessions, perhaps using several different browsers. Google tools have no effective way of stitching these sessions together to reveal the individuals behind them.
5 things you need from an attribution solution that Google Analytics can’t provide today’s marketers
For any marketing attribution tool to be useful, it has to start by cleaning up the raw data, to give a more accurate picture of the true customer journeys behind the digital data. Using a combination of regression analysis and probabilistic modelling, QueryClick’s Corvidae, a software-as-as-service (SaaS) marketing attribution tool, uses a proven Random Forest algorithm in a machine learning process to rebuild the raw, cross-channel analytics data. The tool then unifies online and offline data before applying neural network processing calculate attribution accurately.
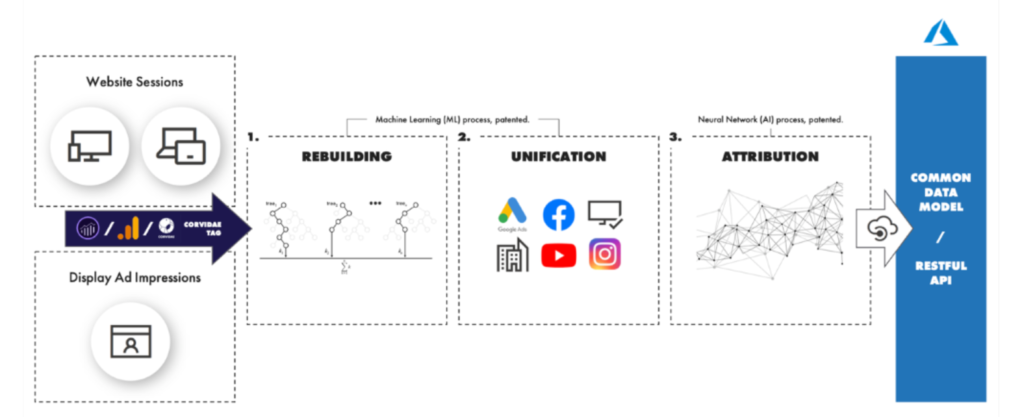
Marketing attribution analysis is never going to be 100% accurate because much of the data that we have to analyse is probabilistic. However, error-ridden, over-simplistic attribution tools are costing advertisers billions of pounds in wasted investment each year. There’s an urgent need to reduce expenditure on running the wrong campaigns in the wrong channels. Without AI, accurate analysis is simply not possible. With it properly applied, the return-on-investment in marketing can be dramatically changed for the better.
‘The Complete Guide to Marketing Attribution’ explains the issues outlined above, and more, in greater detail. Download it below.
The Complete Guide to Marketing Attribution
Own your marketing data & simplify your tech stack.
Have you read?
Chrome’s announcement on dropping cookie opt-in last month closed the door on a 5 year saga for marketers. But what is the landscape like in 2025 for cookie-based measurement?
Generative AI is transforming the way that marketers plan and assemble content for their Paid Ads. As big platforms like Google, Meta and TikTok increasingly build the tools needed to...
In a surprising move that has sparked heated debate, Mark Zuckerberg announced on his Instagram that Meta will be reducing its levels of censorship and in particular fact-checking on its...