Machine Learning and Digital Marketing
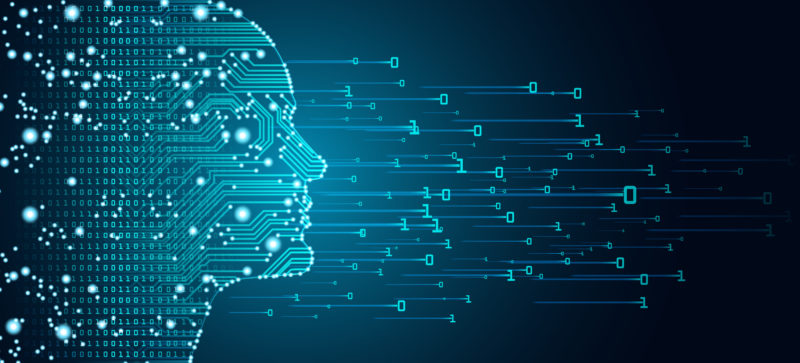
As technology continues to develop and evolve, the way in which we operate our digital marketing also needs to change. Taking advantage of these technologies can not only enable efficiency but can also unlock insights that would be difficult to do using traditional manual processes.
Using Machine Learning to take your digital marketing to the next level will become increasingly important over the next few years. But knowing its uses and where to begin can be a challenge.
In this article, we will provide you with a few starting points, including:
- What is Machine Learning?
- Machine Learning in SEO
- Machine Learning in Paid Search
- Machine Learning in Customer Analytics and Attribution
What is Machine Learning?
While Artificial Intelligence (AI) and Machine Learning (ML) are sometimes used interchangeably, Machine Learning is a subset of Artificial Intelligence that focuses on using computer algorithms to automatically improve through experience.
What does this mean in layman’s terms? Essentially, ML algorithms use a sample set of base data to make decisions or predictions without ever explicitly being told to do so.
AI and Machine Learning in Digital Marketing
Machine Learning and SEO
Machine Learning has, in fact, been a part of SEO for a while now.
Back in late 2015, Google confirmed the existence of RankBrain, the machine learning element of its core algorithm. The purpose of RankBrain is to help Google gain a deeper understanding of what the right search result to serve users really is.
Prior to RankBrain, Google used its basic algorithm to determine which result to show for a query based on the traditional algorithmic signals. In a post-RankBrain world, Google applies machine learning to try to ascertain the true intent behind a user’s search – as a result, Google believes it will provide a more accurate service.
To do this, Google analyses behavioural user data to help understand what a user is searching for beyond just the words that are typed in.
This ties in very closely with Google’s Hummingbird algorithm that was used to expand Google’s semantic understanding of search queries. With both running simultaneously, Google is looking to build a truly intent-based, semantically understood search result for all queries. Without the use of machine learning, this is a near impossible task.
This process looks to include another form of artificial intelligence – Natural Language Processing (NLP), which is designed to allow computer systems to not only read human langue, but also be able to decipher it and truly understand the context behind the words.
We see this in tools such as Grammarly, which uses NLP to understand what a user is trying to say in their text and make grammatical recommendations to improve it. Without NLP, the product simply wouldn’t work.
What does this mean for SEO?
It’s pretty simple: ensure that your content is created with users and their intent in mind.
While keywords are still important to help inform search engines what pages are about (page titles etc.), ensuring the content meets user expectations for the search terms you desire is vital.
Thematic content clusters are the future, and the “one page for one keyword” mindset should well and truly be a thing of the past.
Machine Learning and Paid Search
Like SEO, Paid Search has been impacted by the advances in ML.
Paid Search marketers make decisions routed in the data from their campaign activity to make enhancements and drive more revenue for their clients/business.
ML has helped digital marketers remove some of the manual data handling elements that used to make the discipline very time intensive.
Bid management was the foray into the ML world for Paid Search – and it continues to be used to this day.
Manually checking thousands (and potentially more) data lines to make individual bid adjustments was a burdensome task – through the creation of bid-automation, Paid Search managers were able to be fed this information through machine learning platforms and make strategic decisions to apply the bids into their accounts.
This is a market that Google themselves have ventured into with Search Ads 360, and there are additional third-party tools on the marketplace too.
Identifying which tool is right for you can enable you to reach far improved levels of not only efficiency, but Paid Search performance via ML insights.
Strategy is still key to performance
While MaL has introduced substantial efficiencies into our SEO and Paid Search activities and execution, the human element of strategy is still key for success.
As with all ML, the initial input data acts as the catalyst for the learning. If this is poor, then the machine will be learning to perform efficiently but poorly.
Therefore, maintaining strategic human input and oversight is key to continued success within both these channels and should remain at the forefront of digital marketing investment.
Machine Learning and Customer Analytics
We live in the data-age. Data is not only King, it’s everything. Without it, many of our digital marketing activities would either not work or be incredibly sub-par in effectiveness.
Yet despite this, we continue to see marketers using poor-quality data or using data inefficiently when trying to make intelligent decisions.
All businesses should have some sort of analytics platform in place if they‘re operating digitally.
Now whether that’s Google Analytics (free of 360), Adobe Analytics, or some other platform, collecting data is only the beginning.
What you do with it is what adds the value and allows you to outperform your competition.
Manually interpreting data is fine, on a small scale, but once you’re having to process large amounts of data it becomes nigh-on impossible.
Applying ML to your analytics will allow you to identify true insights, which in turn lead to real-life recommendations designed to improve performance of your site – and in the long term, drive increased revenue and ROI.
How Corvidae uses Machine Learning
At QueryClick, we identified inaccurate attribution as a core challenge for digital marketers and decided to solve it. Attribution has long been a sticking point for many companies, not knowing if, or where, the money they invest across their marketing channels is really having an impact.
On top of this, fundamental flaws with data collection and processing by traditional platforms means that a significant portion (up to 80%) of your analytics data may be incorrect or missing. Our proprietary software, Corvidae, doesn’t just analyse your data – it rebuilds it from the ground up.
In order to rebuild your data, we use machine learning techniques to “see” the individuals behind the clickstream data and understand their full customer journey on an individual level.
We do this to understand where users are truly coming from to be able to plug the Direct/Other traffic source gaps often found in off-the shelf products.
All of this provides us with what we call Visit-Level Attribution: the ability to see every visit by every user.
This improves the quality of data that you’re then able to use to inform your campaign activation.
Corvidae also provides you with attribution data you can trust to make intelligent investment decisions into the channels that drive the most value for your business.
In Conclusion
Machine Learning has fundamentally changed the way search engines understand content and the way their users are searching for information. Marketers need to be more intelligent in how they create our content to ensure they satisfy the thematical and intent basis behind the keywords, and not just the keywords themselves.
Additionally, ML allows us to drive efficiencies in our Paid Search activity and allow our Paid Search marketers to focus on strategy, not just number crunching.
Finally, through Machine Learning digital marketers are able to derive more insight from our analytics than ever before.
Want to know more?
Chat to us about how you can incorporate ML into your marketing strategy.
Own your marketing data & simplify your tech stack.
Have you read?
Chrome’s announcement on dropping cookie opt-in last month closed the door on a 5 year saga for marketers. But what is the landscape like in 2025 for cookie-based measurement?
Generative AI is transforming the way that marketers plan and assemble content for their Paid Ads. As big platforms like Google, Meta and TikTok increasingly build the tools needed to...
In a surprising move that has sparked heated debate, Mark Zuckerberg announced on his Instagram that Meta will be reducing its levels of censorship and in particular fact-checking on its...