A Beginner’s Guide to Marketing Attribution
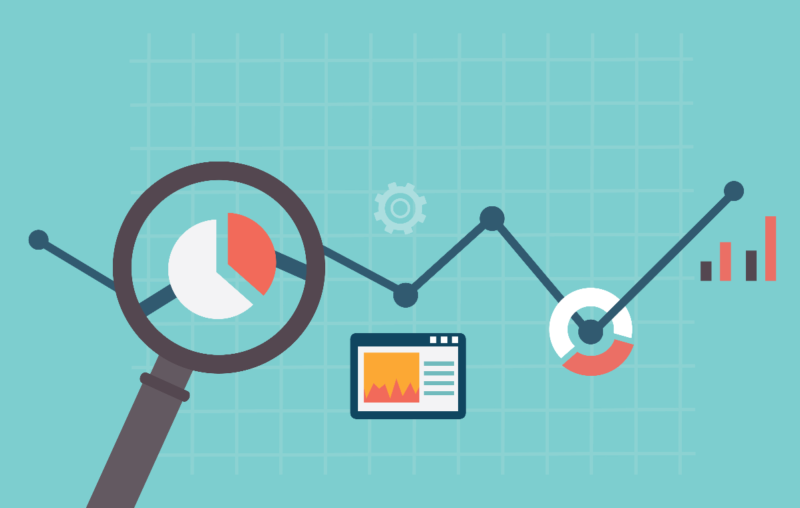
Many discussions about marketing attribution, like other aspects of marketing, are full of jargon.
Most articles on the subject get complicated quickly, and by the time you get to paragraph three, it’s easy to have forgotten the meaning of some new terminology mentioned in paragraph one.
So, this blog post is an attempt to cut through the industry patter and to explain, in plain English:
- What marketing attribution is
- Where it came from
- Its current state of development
- Where it’s going
In that context, we’ll take a brief look at artificial intelligence and the role it has to play in this game.
What is marketing attribution?
Marketing attribution is the process of allocating value to marketing interactions with customers. The value is usually expressed in monetary terms. Marketing interactions may be seeing an advertisement on the back of a bus, looking through your website, reading an article about you in the press, placing an order, or a myriad of other things. Marketers call where these interactions take place ‘touchpoints.’ Customers may be current, past, or future and if they do what you want them to do as the result of an interaction, which may be anything from visiting your website to placing an order, that’s usually referred to as a ‘conversion’.
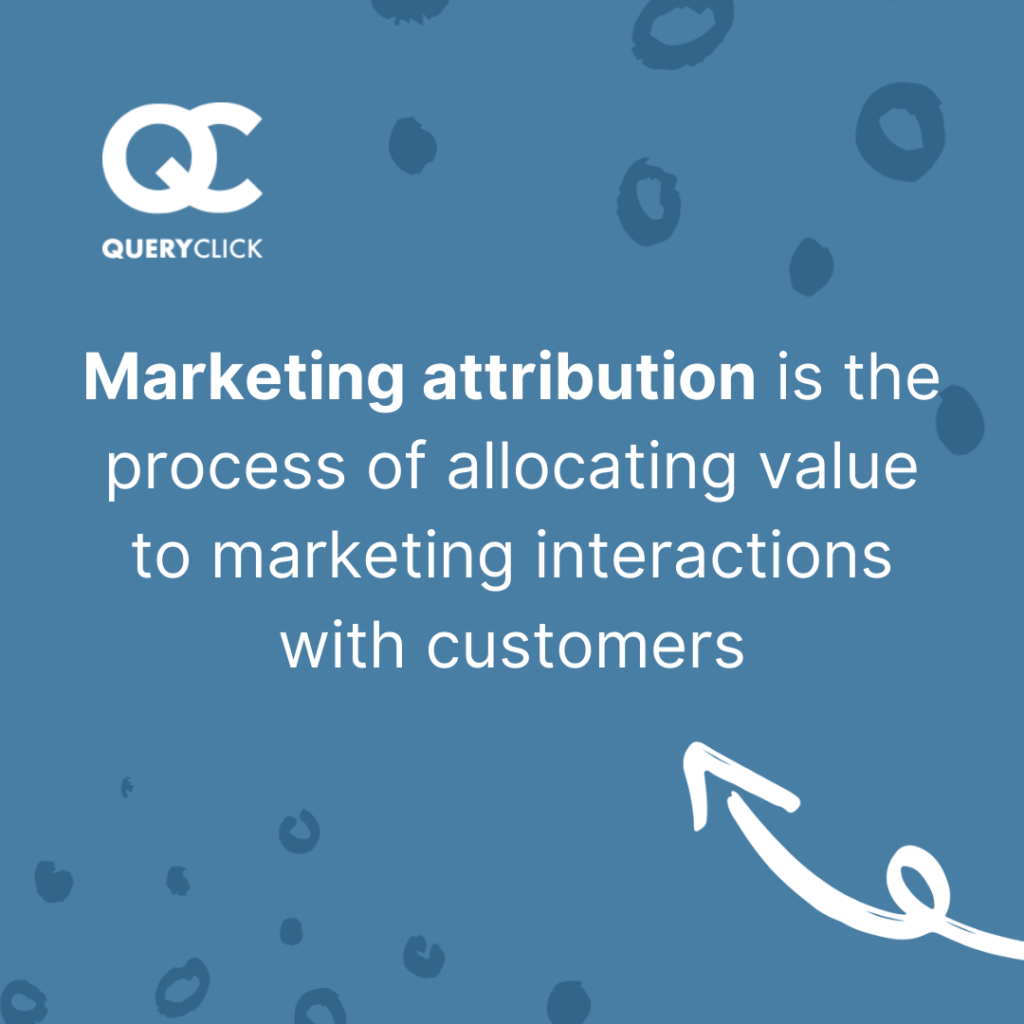
The primary aim of most marketing activities is to persuade customers to buy from you so that you can grow revenue and profits. Incidentally, there is often too much emphasis on revenue when it would be better to focus on profits. It may be a little more complicated to calculate the impact of marketing on profits vs. revenue but a marketing plan designed to maximize revenue may look different from one for maximizing profits.
This article by Avinash Kaushik goes into more depth on the subject.
To allocate your marketing budgets to the best effect, you need to figure out how much each marketing interaction influences the bottom line. Data may be produced for each touchpoint, but the type of data will vary, depending on the nature of the interaction. Then there’s the question of how marketing interactions affect each other. Marketers call this ‘incrementality.’
An incrementality example
Let’s say you want to buy an espresso coffee maker.
You head over to the Amazon website to see what’s available and encounter a choice of machines from brands that you recognise – Nespresso, De’Longhi, and Sage. But you also see a machine from Swan, a brand you don’t know.
The Swan machine is cheaper, and its reviews are reasonable, but there’s still that nagging doubt because the brand is new to you.
You have no ‘feel’ for the brand and it’s a lot of hassle to have to pack up a coffee maker and send it back if you have a problem. So, you choose the Sage machine and place your order.
Back at Sage, the marketing manager sees that his advertisement on Amazon has led to a sale. But should he attribute the value of the sale solely to his investment in the Amazon ad? Certainly not.
You made your purchase based on the confidence you have in the Sage brand, and that didn’t come from the Amazon ad. It may be that you saw the product in retail stores, in a TV or magazine advertisement, or even just in a friend’s kitchen, but it was other interactions with the Sage brand that gave you the confidence to believe that you were making a good purchase.
The marketing manager’s challenge is to figure out which other interactions with the Sage brand built that confidence.
For example, they may want to understand whether their investment in TV advertising influenced your decision, and what incremental difference it would make to Amazon sales (or overall sales) if they cut TV advertising altogether.
This is the issue of incrementality. It is one of the greatest challenges facing marketers because there are so many communications channels to choose from, so many ways to combine those channels, so many different creative strategies that could be deployed, and all of this in a constantly changing competitive environment.
Related: [Blog] What is Incrementality? (And How to Measure It)
Where attribution started and how it developed
Coupons – a real breakthrough
The idea of marketing attribution is at least 100 years old. When describing advertising in 1923, copywriter and author of the book ‘Scientific Advertising’, Claude Hopkins, said,
“The causes and effects have been analyzed until they are well understood. Advertising, once a gamble, has thus become, under able direction, one of the safest business ventures.”
Although undoubtedly a genius of his time, Hopkins was dealing with a much simpler world. He is credited with inventing coded coupons that customers took into shops when making purchases. All marketers had to do was count the coupons from a particular campaign to calculate the cost-per-purchase of their advertising. They could easily compare the effectiveness of different media outlets, mainly magazines and newspapers, and test different creative treatments to see which were most effective in terms of response. Hopkins was a committed direct-response advertiser, a direct marketer who saw little or no value in ‘brand’ advertising.
Media mix modelling – progress, of sorts
As marketing channels became more varied, particularly with the advent of radio and television, there was a need for a more sophisticated approach to evaluation. Originally developed in the 1950s, the idea of media mix modelling (MMM) came into vogue in the 1960s – 70s. This involves:
- Taking marketing data from multiple sources
- Cleaning it up
- Applying statistical analysis to extract high-level insights about the impact of various channels and campaigns.
In its day, it was advanced stuff but the advent of multiple digital channels, including social media, has undermined its usefulness because of the limited amount of detail it reveals. The degree of detail in marketing data (or any other data) is often called its ‘granularity’.
Last-touch attribution – and its penalty
Around the end of the 1990s, the concept of last-touch attribution came to the fore, driven by Google Analytics tools and later, Adobe Analytics. Despite later advances, which we’ll come to, last-touch attribution, which assigns all the value of a sale or other type of customer conversion (customers taking the desired action) to the last marketing interaction they had with your brand.
When you think about it, this is not so different from collecting coupons, as marketers did 100 years ago, but it is not true attribution because no other marketing activity is measured or valued. Nevertheless, this approach is alive and well today and is still used by most marketers.
But here’s the catch. If you only value the last touch, the most impressive results are likely to come from pay-per-click advertising, your ads appearing as a result of someone typing relevant words into a search engine.
Because the results are immediate and easily measured, your competitors follow suit and focus their budget on pay-per-click advertising.
But the supply of the advertising slots that appear in response to particular searches is limited and in Europe some 80% of pay-per-click advertising is dominated by two channels, Google and Facebook.
As a result, the cost-per-click is going up all the time, and the cost for you to achieve the desired response from a customer is rising with it.
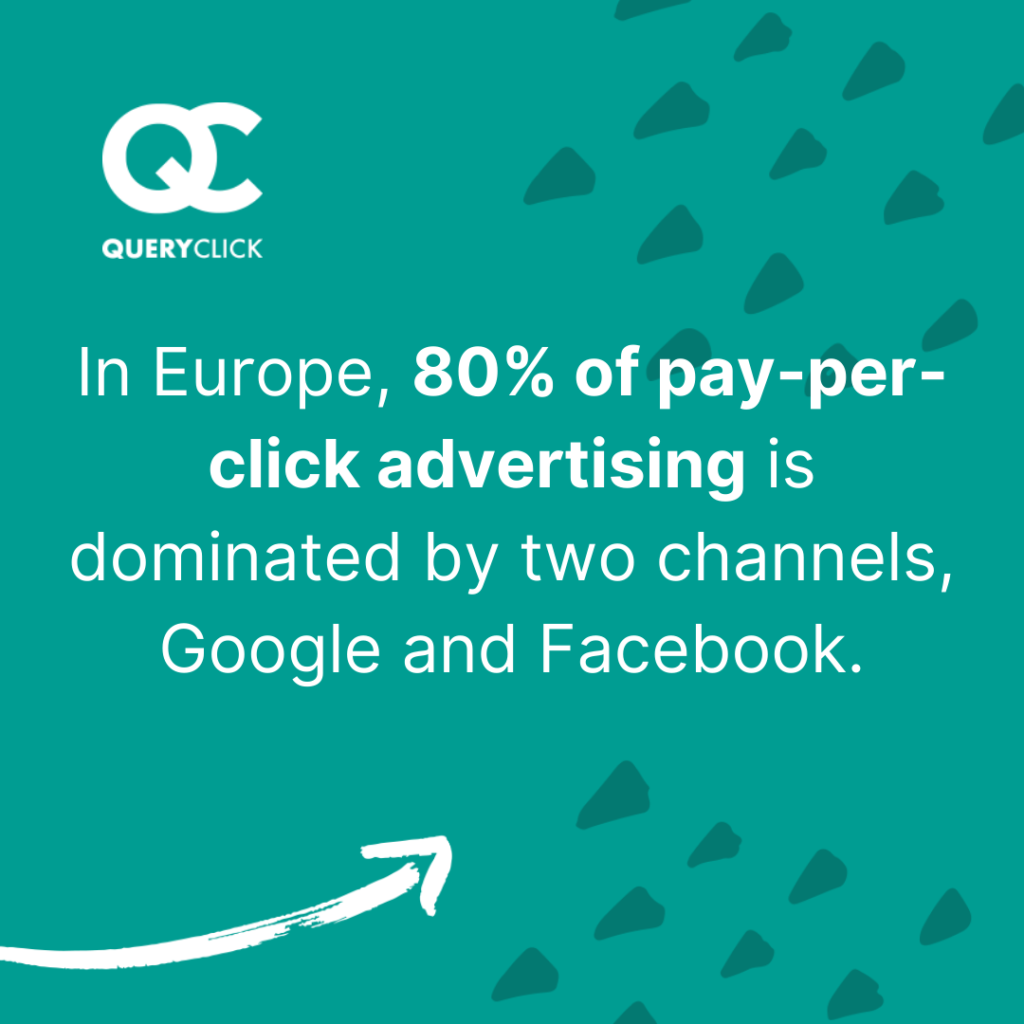
Google Analytics provides data that appears to show Google pay-per-click advertising as delivering the best value for your marketing budget. It ignores the value of all other marketing efforts, online or otherwise. If taken in isolation, you cannot trust the data any more than you’d trust a mouse to design an effective mousetrap.
Rules-based, multi-touch attribution = process + guesswork
From the mid-2000s onwards, marketers began to try rules-based, multi-touch attribution models. This blog post by the Digital Marketing Institute summarises seven of the most popular: linear, time-decay, U-shaped, W-shaped, full-path, weighted multi-source, and custom models.
These models are, like media-mix modelling, an attempt to attribute value to all marketing investments, online or traditional, like print or TV advertising. At first sight, they all seem logical in their approach, but they share a common flaw.
The weighting applied to each marketing interaction, or touchpoint is the marketer’s best guess. If that guess is wrong, so is the output of the model, and so are the marketing investment decisions based on that output.
In the blog post we mentioned earlier, Avinash Kaushik comments,
“How do you get over the frustration of having done attribution modeling and realizing that it is not even remotely the solution to your challenge of using multiple media channels?”
And marketers’ lack of confidence in data doesn’t just apply to marketing attribution. It’s a bigger issue. The latest Marketing Data and Analytics Survey 2020 from analyst firm, Gartner, revealed that “over 50% of senior marketing leaders are unimpressed with the results they receive from marketing analytics investments.”
How to fix broken attribution with a little help from artificial intelligence
I don’t know how Sage’s marketing team does attribution but let’s assume that the company invests in TV and print advertising, email marketing, in-store promotions, social media advertising, and pay-per-click Google ads.
For some of these channels, the data coming back to the marketing department will be clear, unequivocal, and 100% trustworthy. Sage’s marketing team will know who responded to what, how they responded, and when. Depending on what data is collected, they may know the following the customer or prospective customer:
- Age
- Gender
- Interests
- Previous purchasing history
This kind of data is called ‘deterministic’.
From other channels, the data will be less clear and certainly not deterministic. For example, some groups of customers are likely to see TV advertising that’s run during sports events, and the demographics of magazine readers are usually well-defined so you can target campaigns towards these groups.
The data that comes from social media networks is not deterministic either, primarily due to the need to protect user privacy. Social media channels are often described as operating ‘walled gardens’ in terms of the limited access to data offered to advertisers.
But you can target your advertising to profiled groups of individuals in the networks and if you suddenly see a surge of orders from individuals that match the profile, your advertising was probably seen by them and affected their subsequent actions.
Using mathematical modelling, you can calculate just how probable, so this kind of data is classified as ‘probabilistic’. The mathematical modelling is done most effectively by applying artificial intelligence, or AI, to the task.
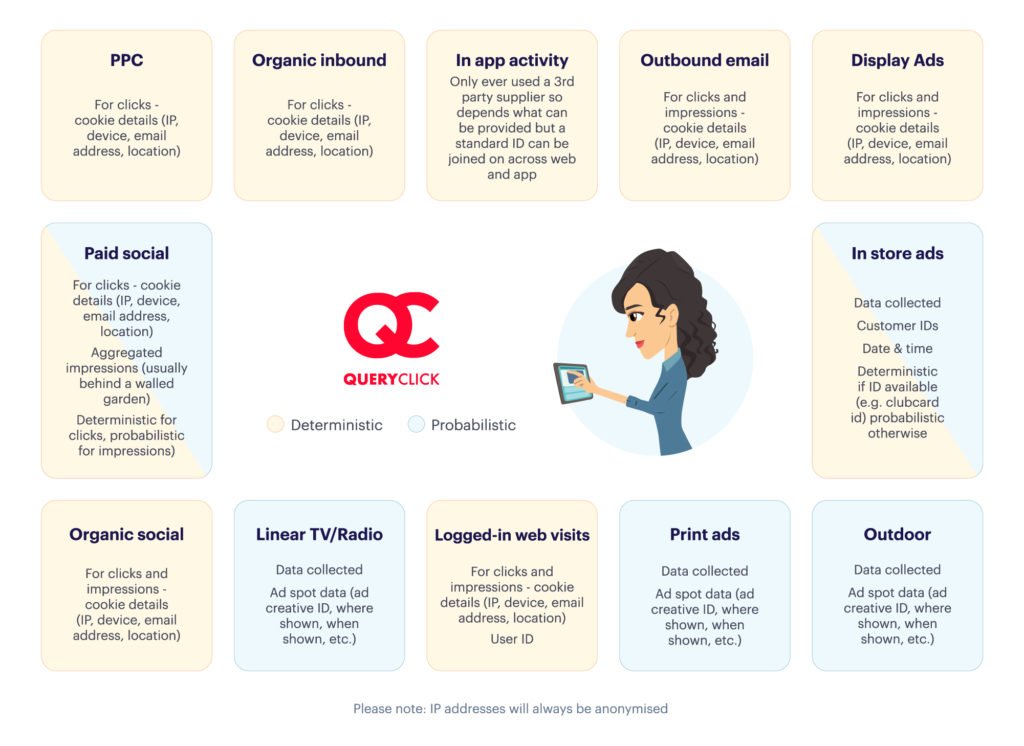
AI algorithms classify and group things. They can process vast volumes of data at high speed and, when properly used, create valuable insights for marketers.
In the context of marketing attribution, the algorithms can be applied to historical data to seek out patterns in customer behaviour. They can be used to calculate probabilities in data that is not deterministic with a degree of accuracy that makes rules-based attribution guesswork look like something out of the Dark Ages.
And, perhaps cleverest of all, they can be used to extract incredibly detailed insights into customer behaviour by merging deterministic and probabilistic data. So much so, that marketers can use these insights to predict future customer behaviour, rather than just look back on it.
Two more vital considerations
There are now perhaps 100 or more companies offering marketing attribution software, either as local or cloud-based tools. Some of their jargon-filled websites and sales literature are impressive but they often mask two fundamental issues.
- Funnelling data from many sources to produce nice-looking charts and graphs to show your boss is not in itself a solution. Recent research has shown that up to 80% of the raw data commonly used in marketing attribution is inaccurate. No amount of combining it with other data is going to change that.
- Much of the data provided by online analytics tools are based on browser cookies and pixels. This means that you’re analysing browsers, not the individuals behind them. One browser may be used by several individuals, and one individual may use several browsers. This limits the value of such data to marketers.
The light at the end of the tunnel
The staggering number of variables involved in measuring people’s exposure and response to advertising or other promotional content means that useful analysis of multi-channel campaigns simply cannot be achieved without using AI, notably in the form of machine learning, or ML. But the data that goes into the machine must be accurate in the first place, so it invariably needs to be cleaned up.
A process that has proven its value is shown below:
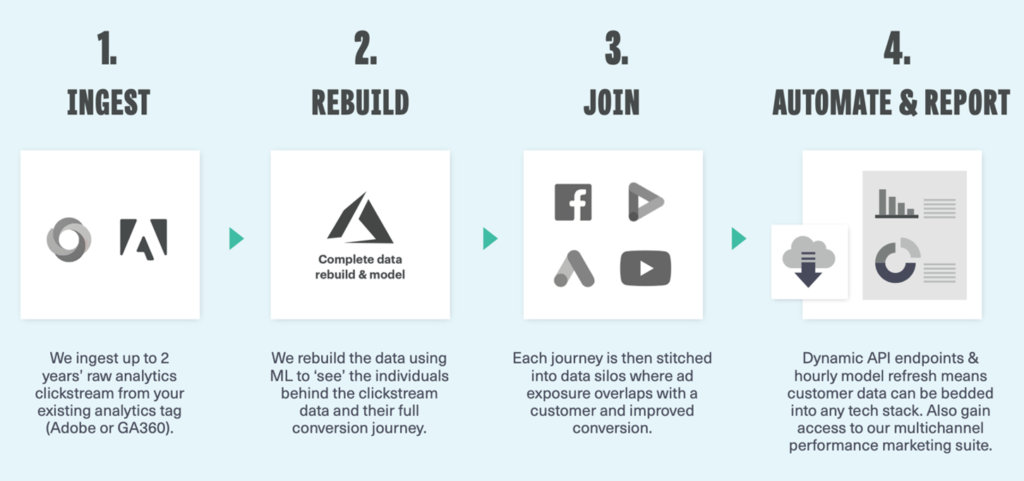
This approach can be applied to look at marketing investment at several levels:
- Channels,
- Campaigns
- Impressions
- Visits
Visit Level Attribution is the latest evolution of the process. It maps and scores customer interactions with each piece of marketing collateral and reveals every piece of marketing’s effect on an individual’s probability to behave in a particular way.
Key Takeaways…
Today’s marketing channels are complex, diverse and often siloed. Marketers are swamped with data in many different forms, some of it deterministic and some of it probabilistic. Only through the smart application of data cleansing and artificial intelligence can this wealth of data be processed in a way that delivers useful marketing insights.
A final thought. If you implement a marketing attribution platform well, the return on investment could be anything up to 40:1. It’s an exceptionally low-risk investment.
The Complete Guide to Marketing Attribution
Own your marketing data & simplify your tech stack.
Have you read?
As you will have likely seen, last week Google released the March 2024 Core Algorithm Update. With it, comes a host of changes aiming to improve the quality of ranking...
After a year of seemingly constant Google core updates and the increasingly widespread usage of AI, the SEO landscape is changing more quickly than ever. With this rapid pace of...
Given the fact that Google has recently blocked third-party cookies from 1% (or 30 million) Google Chrome users, and their entire removal is expected by the end of Q3 of...