Four of The Most Expensive Mistakes in Marketing Attribution
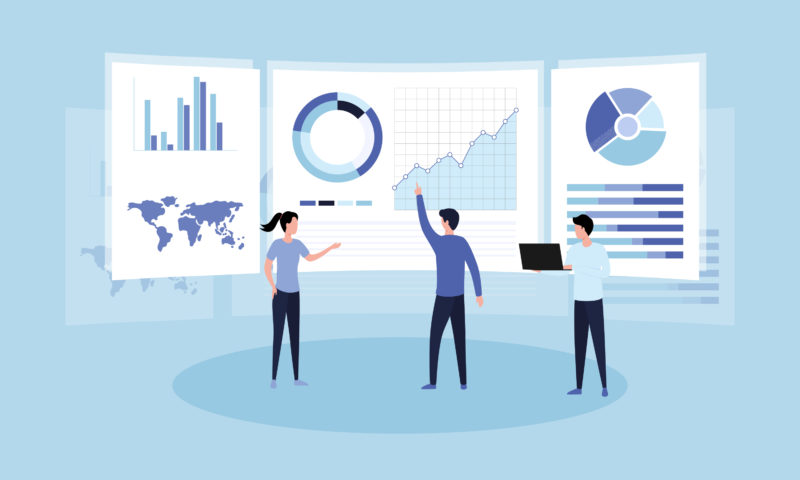
Marketing attribution is the process by which companies try to figure out which marketing efforts are most effective in driving sales. But can it be done effectively, given the volume and complexity of data with which marketers are bombarded?
This article takes a fresh look at the challenge and how some of the common errors made by marketers may account for their dissatisfaction with attribution outcomes.
A quick overview of attribution
Marketers everywhere are under growing pressure to prove return-on-investment (ROI).
On the face of it, this might appear simple: if you spend more on advertising and promotion, do sales increase and, if so, by how much? And in this digital age, marketers are blessed with an abundance of data from which they can extract the much-needed evidence that their budgets are being invested well. If only it were that simple.
The 19th century Philadelphia retailer, John Wanamaker, is said to have exclaimed, “Half the money I spend on advertising is wasted; the trouble is I don’t know which half.”
But it was not that long after, in 1923, that copywriter Claude Hopkins declared in his book “Scientific Advertising”, “The time has come when advertising has in some hands reached the status of a science. It is based on fixed principles and is reasonably exact. The causes and effects have been analysed until they are well understood.”
Hopkins was an advertising pioneer upon whose work much of today’s advertising is based and he revolutionised the measurement of ROI in his day. But the limited number of available media channels, and the deterministic nature of the data he collected – customers walking into a shop with numbered coupons on their hands – made it simple to analyse the results of individual advertisements and campaigns.
Incidentally, Hopkins was not concerned with brand advertising in its wider context. He was a firm advocate of what we now call direct marketing (or direct-response advertising) and in 1907 he attracted a salary of $187,000 a year for his pioneering work in the field. Adjusted for inflation, that would be around $5 million today.
Today’s marketers face a greater challenge in proving the ROI of marketing budgets. They are drowning in data from myriad channels, and much of that data is siloed and mysterious. A 2019 survey of digital marketing professionals in UK retail, travel, and finance businesses found that 61.5% of respondents agreed with the statement that “Investment strategy decisions we make based on attribution insights generally fail to deliver the predicted results.”
Despite the promises of attribution software vendors, attribution is broken. Or perhaps it has never worked in the first place.
Here are four of the most common causes of attribution errors.
The most common attribution mistakes
Mistake 1: Over-reliance on last-click attribution
Most marketers rely on ‘last-click’ data and only attribute marketing value to the activity that produced the click.
Often, this is pay-per-click (PPC) advertising. Why does this happen? Because PPC is controllable, produces immediate results, and it’s easy to measure conversions.
To the naïve marketer, it looks like an easy way to demonstrate ROI. To a smart marketer, it’s not demonstrating attribution at all. If it were, why would you bother with any other marketing activity except PPC?
When trying to understand where marketing investment is most effective in creating sales conversions, last-click attribution is illusory.
Mistake 2: The belief that rules-based, multitouch attribution is any better
There are several well-established models for multi-touch attribution.
They include:
- last-touch
- first-touch
- linear attribution
- time-decay
- U-shaped and W-shaped attribution
- full-path attribution
This blog post on the G2 Community goes into more detail on most of the models.
The major flaw with these rules-based models is that the values assigned to each touchpoint are subjective. For example, in the U-shaped model, it’s usual to assign 40% of the value of a conversion to the first click and 40% to the last, spreading the remaining 20% over the points in between. Why? Where do these percentages come from? They are just guesswork. Even if they’re accurate for one set of circumstances, they won’t be for most others
Mistake 3: Failure to grasp the complexity of marketing data, particularly when trying to combine offline and online streams
Most marketing analytics are based around cookie and pixel data. Cookies are supposed to help you join up multiple sessions, to help you understand more about the buyer journey. But even this simple concept is deeply flawed.
A browser may be used by several individuals in the same household or office. You have no way of knowing who created a specific session, even if you’ve captured an IP address and the device user agent data (OS and device type). Your customer may use browsers on a variety of devices: computers at home and at work, smartphones, and tablets, all while researching a purchase.
Some buyers will have their browsers synchronised across the devices they own, others will not; some may be savvy enough to regularly clear down cookies or use their browsers in ‘private’ mode specifically to avoid being tracked.
Social media, both earned and paid, obviously has a big role to play in most campaigns, and the data from these individually siloed channels also needs to be considered in any meaningful analysis of marketing attribution.
Marketers also want to understand what prompted customers to engage with them in the first place. This could be some offline activity, perhaps TV, radio, or print advertising. It could even be through earned media that was published in print some time ago. Here, the data is not deterministic. You can’t be sure that a given customer saw your message in a particular channel.
That said, you might assume that if you ran a successful TV campaign within specific region and attracted a lot of new visitors to your website from that same region, there is a reasonable probability that many of them were influenced by your campaign. And a deep understanding of probabilistic modelling, and how it can be usefully and meaningfully combined with deterministic data, must therefore be at the core of any credible marketing attribution platform.
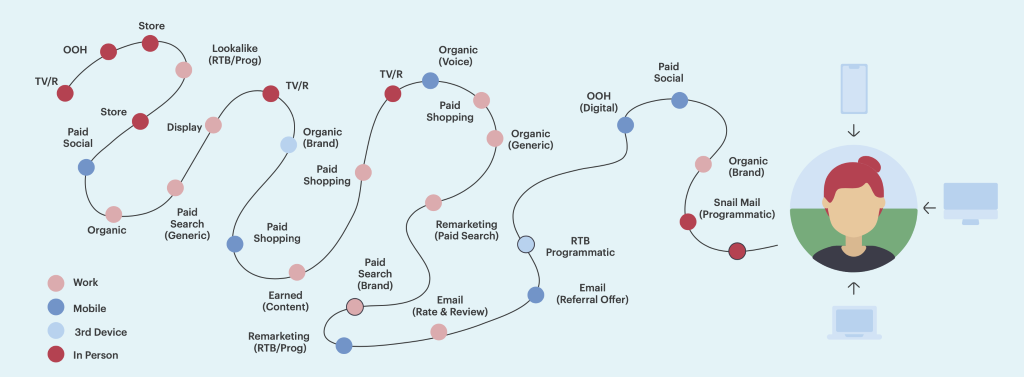
Mistake 4: The assumption that simple data consolidation helps with attribution
At last count, there are now more than 40 companies promising to deliver better attribution through online software platforms. They range from giants like Nielsen and IBM to smaller businesses, some of which only address niche markets.
From the generic promises made on their websites or even their supporting marketing materials, it can be hard to figure out exactly what’s being offered.
A lot of them are simply promising to make things easier for marketers by connecting data from a lot of diverse sources and presenting the merged results in nice-looking charts.
The problem with this approach is the contamination of good data with bad. If you funnel just one error-ridden data source into the mix, the overall result is badly compromised.
In practice, few data sources are without error. Bots pretending to be customers, publishers massaging data to generate higher revenue, privacy legislation occluding customers from marketers, and Google tweaking its algorithm thousands of times each year are just some of the daily challenges of achieving data accuracy.
And that’s before you consider how to measure the effects of different creative approaches in advertising or the impact of competitor activity
How machine learning creates a light at the end of the tunnel
According to analyst Markets and Markets, the market for marketing attribution software will have grown from USD 1.8 billion in 2018 to USD 3.6 billion by 2023 at a compound annual growth rate of 14.4% during the forecast period.
This scale of opportunity is attracting a lot of snake oil and if marketers don’t work hard to understand what they’re buying, they could end up wasting as much on attribution tools as they already do on advertising.
Marketers have access to more data than they could have dreamed of even just a decade ago. But data has become more of a problem than an asset. Some is deterministic – you can identify the source and the customer intent behind an action. But much of it is probabilistic and subject to hundreds of variables. It’s hard to see the wood from the trees.
You can’t just combine data sources and hope to create meaningful marketing insights. This is where machine learning (ML) has a vital role to play. It’s a form of artificial intelligence (AI) or, as some people refer to it, ‘augmented intelligence’. ML algorithms make light work of the statistical analysis required to understand probabilistic data. They reveal patterns in data that it would be difficult or impossible for humans to see and enable streams of data to be broken down and rebuilt from scratch. Only then are deterministic and probabilistic data merged to create accurate, meaningful attribution insights.
Without ML, attribution is up to 80% inaccurate, leading to massive wastage in marketing budget allocations. Properly applied, the technology can connect single customer journeys across numerous online and offline marketing channels.
This creates individual 360-degree customer views that reveal over 3X more data for attribution than conventional rules-based approaches. This means that marketers can then, for the first time, properly value top-of-funnel brand activity and non-converting content and impressions.
For more detailed information, QueryClick’s 14-page ‘Guide to Marketing Optimisation’ can be downloaded here.
Own your marketing data & simplify your tech stack.
Have you read?
Chrome’s announcement on dropping cookie opt-in last month closed the door on a 5 year saga for marketers. But what is the landscape like in 2025 for cookie-based measurement?
Generative AI is transforming the way that marketers plan and assemble content for their Paid Ads. As big platforms like Google, Meta and TikTok increasingly build the tools needed to...
In a surprising move that has sparked heated debate, Mark Zuckerberg announced on his Instagram that Meta will be reducing its levels of censorship and in particular fact-checking on its...