How Will the Removal of Third Party Cookies Impact Attribution?
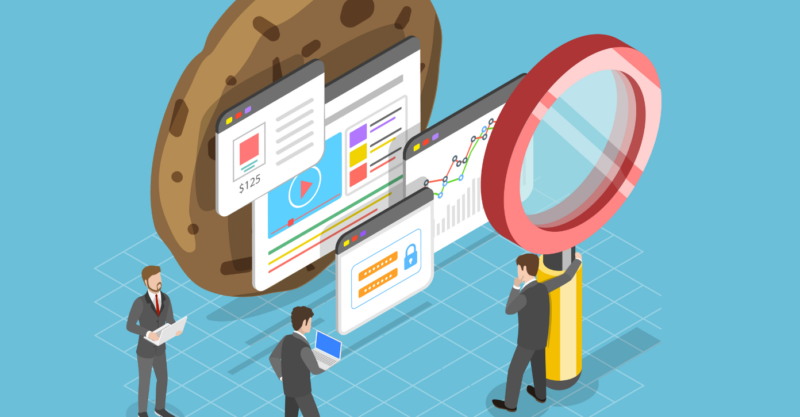
There has been plenty of recent heat around 1st and 3rd party data usage given the impending ‘cookie apocalypse’ that will be triggered by Google removing 3rd party cookies from Chrome in the very near future.
In case you are unaware of how big a deal this is, here is Chrome’s marketshare which, along with the second largest user-agent – Apple’s Safari – will be removing their support of 3rd party cookies by default.
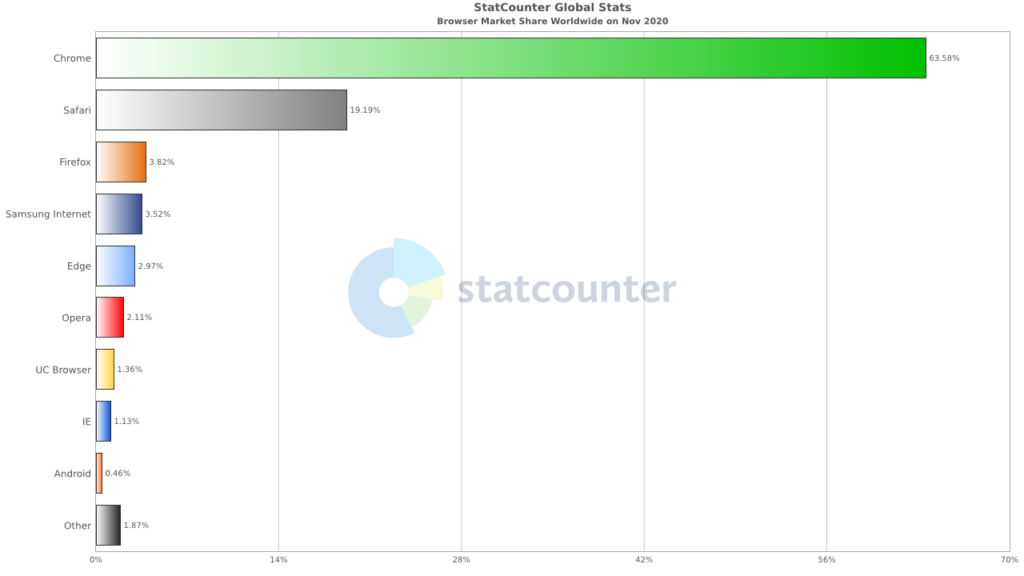
What are 3rd party cookies used for in marketing?
3rd party cookies are typically bought to buy ad inventory against by AdTech and the largest of those networks is Facebook, who has kicked up a fuss about losing their ability to track Facebook users across the open web at more or less the same time as it loses access in-app to their ad interaction data thanks to Apple’s replacement of IDFA with App Tracking Transparency in iOS14.5.
However, lost in much of the discourse is the implication for ‘Reach’ for marketers – reach is shorthand for the ability to place ads in front of large audiences.
This is the main reason that a market exists for 3rd party cookies at all and why there has been such huge growth of Google and Facebook Ad revenues – they own the majority of the web audiences between them.
But there is a larger discourse to be had about the reality of reach for digital marketing channels, as research suggests that the actual reach of the vast majority of campaigns is really measurable in the order of tens or hundreds of websites, rather than the implied hundreds of thousands that programmatic vendors would have marketers believe.
And there is also fairly compelling research evidence that increased targeting using 3rd party cookie audience data reduces the effectiveness of performance marketing campaigns, rather than improves it.
So what to do for today’s marketer in such a changing, uncertain system?
In this post, I aim to summarise the options available for 1st party audience optimisation and how to understand and maximise reach for your digital multichannel campaigns.
How to lessen the impact of third party cookie loss
Step 1: Maximise your 1st Party audiences
Ensure containerisation & tag management best practice
1st party data is data generated by a pixel served directly by your own server. It is possible to embed pixels from 3rd party suppliers as 1st party using a process called ‘containerisation’ for either the tag manager, or your server infrastructure using, typically, Docker or Kubernetes.
I encourage as a first step ensuring all pixels served by your site’s tag manager use containerisation to ensure 1st party compliance – and it goes without saying you should ensure you have clear, required data use policies for opting in or out for your pixel’s cookies.
Secondly, utilising custom user stitching with your analytics platform of choice is now non-optional for effective 1st Party data generation and enrichment.
We adopted Snowplow many years ago at QueryClick and have found it to be excellent and robust to enterprise class deployments capturing hundreds of millions of rows of data a day with no issue what so ever using an Azure deployment environment.
Apply data cleansing and rebuild broken cookie data
It’s pretty well documented now that relying on cookies – even 1st party cookies – for any kind of session stitching is a fools errand.
Making your custom session stitching hook into a service like Corvidae is key to allowing you to correct the 80% incorrect data returned by Google and Adobe Analytics – and indeed Snowplow.
The reason for this is simple: sessions are a binary measurement in a messy world.
Consider your own habits when going through the process of, say, purchasing a pair of shoes.
There is a phase before you become consciously aware that you would like to buy some new shoes. This ‘pre-consideration’ phase is typically what is being targeted by brands with ‘brand marketing’ activity.
Think about Nike, or Birkenstock – you already have a picture in your mind of what those brands represent and if those are values you hold or – more likely(!) – aspire to, then you may commence your ’Consideration’ phase with searches around their brand terms or specific footwear they have promoted to you – likely via rich ads – video or display – which if constructed correctly will perform better for brand recall if they are shorter – ideally a 15 seconds total ad time with brand shown within 7 seconds.
Most consideration phases which end in a transaction will have a great number of marketing touchpoints even if the conversion time is short. Those touchpoint will not only be distributed across multiple digital and offline channels, but also will feature multiple repeat visits to – for example – the brand website before the conversion is commenced and, barring an exit to another brand, completed.
A session has to bridge all of those channels and all of those repeat visit sessions to offer an accurate picture of the human behind the devices.
Pixel query parameters and 1st party cookies are a laughably inadequate way to stitch together this kind of modern conversion journey. Which is why we see 80% inaccuracy in ‘unique session’ creation in Google and Adobe – and Snowplow – deployments.
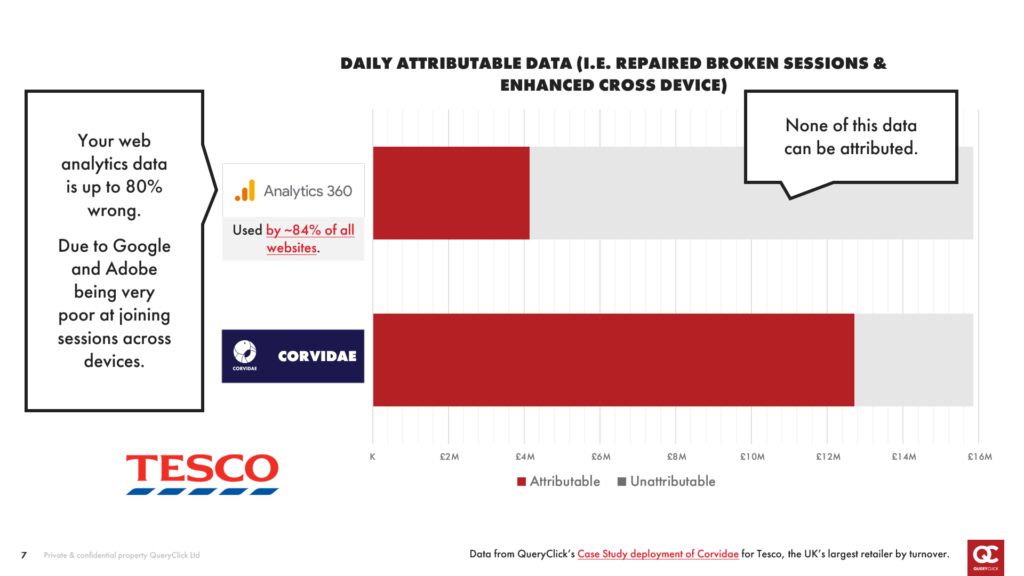
At QueryClick, we have spent 7 years and several millions of pounds developing globally patented approaches to ‘rebuilding’ those broken sessions using machine learning, deep learning, and probabilistic modelling developed in a research partnership with the largest University data science department in EMEA – Edinburgh University – in a compound attribution modelling process that we deploy for our customers to offer radically more accurate and granular attribution data than is available anywhere else in the world today.
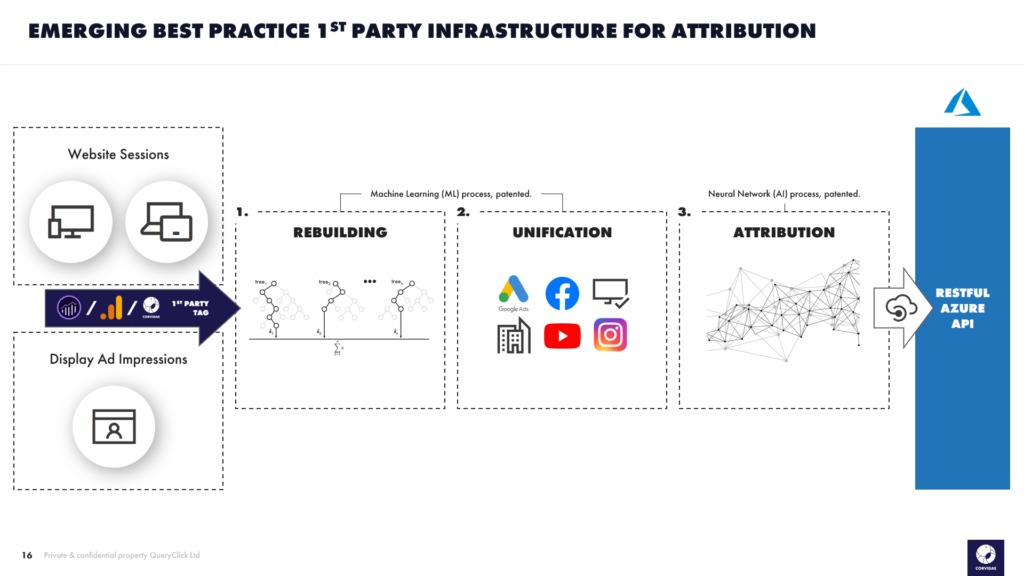
Ultimately, by deploying technology like Corvidae, with your 1st party containerised pixel collection across owned adtech ad slots, you then are obliged to unbundle the siloed marketing data from the dominant AdTech players today who will be influencing your target customer behaviour and who you will be running ad activity with if you are anything like the vast majority of brands – that is:
- Google,
- YouTube (also Google),
- Facebook,
- Instagram (Also Facebook),
- and increasingly Amazon.
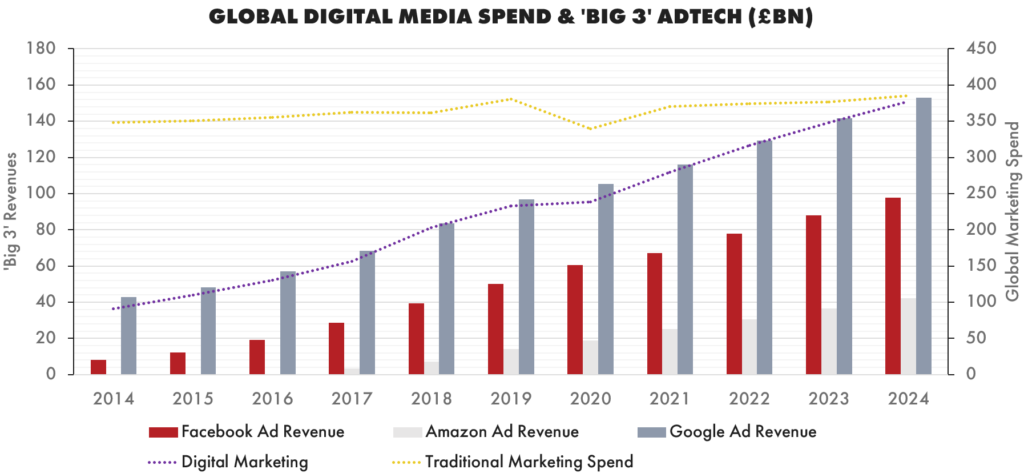
Unbundle Facebook and Google for effective attribution
To achieve that unbundling – as each of these AdTech players have their own 1st party pixel collecting data that you need to somehow integrate into your own 1st party analytics data – you must tackle the largest headache for all marketers today: attribution!
Don’t believe attribution is a problem? Check out the below comparison of the same activity in Facebook compared to web analytics data reporting (both on minimal attribution assumptions – 1-day click and view for Facebook, last click for Google Analytics) for a client of ours:
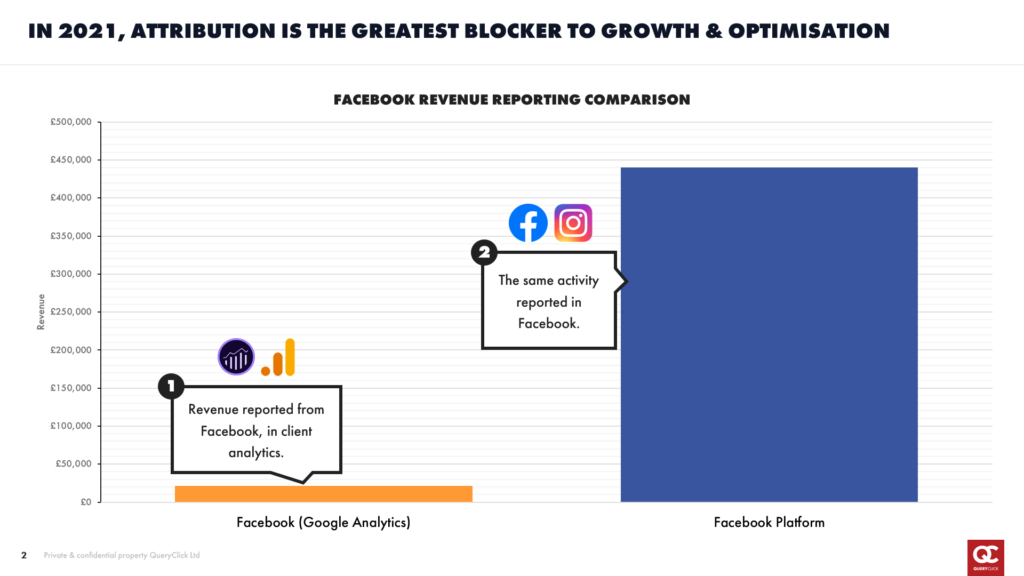
So you tell me what the real revenue number is!
This is the data silo problem that is crippling marketing today, and further balkanisation is expected over the next five years.
The discrepancy in our Facebook activity chart is caused, of course, by Facebook’s desire to value the impressions that are influencing customer behaviour without causing them to click and turn up in your 1st party analytics data. And remembering our example shoe shopper earlier it’s a fair desire – they undoubtedly will be influencing that behaviour.
But by how much?
And where is that revenue to come from – because your click data is attributing that revenue elsewhere right now because ultimately your analytics has to line up with cash in the bank – and for the vast majority of you, you will be using an attribution rule – last click, or basic multitouch – to report on digital revenue into your finance function even though that assume virtually none of the conversion complexity we know occurs ahead of a purchase occurs at all.
How does Corvidae compare to Last Click? Read the blog >
This challenge is why I have been an advocate for probabilistic analytics (Machine Learning, AI) to replace simple deterministic (pixel parameter, cookie data) analytics to enable genuinely detailed and exciting new levels of accuracy and control for marketers on the ground who have to make day to day optimisation decisions.
Adopting ML approaches to data rebuilding is the only practical way to model the complexity of the conversion behaviours we are seeking to interact with as marketers, and when applied well can offer highly robust outcomes – typically Corvidae models that have more than 25 transactions a day to work with, will deliver between 85 and 95% statistical confidence intervals.
I also firmly believe in an open-box policy to our technology: enabling client access to the compound model attributed weighting for individual conversion journeys, with associated confidence intervals, allows radical transparency and the opportunity to demonstrate and build trust in the foundational data set being created by Corvidae’s ML models.
Because ultimately, what is produced when building a probabilistic data set is a completely new ground truth for your marketing activity.
Every stitched together session that crossed multiple data silos including:
- 1st party analytics pixel
- 1st party display ad impression
- Facebook aggregated
- API impression data
- Search Ads 360 Floodlight tag
- TV spot data
- App Install
- ERP Till
- Store Footfall data
For example, I’ve written before about the value and impact of campaign level attribution reporting – something completely unavailable anywhere else in the world currently, and enabled entirely by our patent protected processes that power Corvidae.
For example, this is how our Facebook discrepancy can be resolved into campaign level reports highlighting which campaigns are lossmaking from an attributed ROAS perspective compared to their reported revenue in Facebook’s own platform.
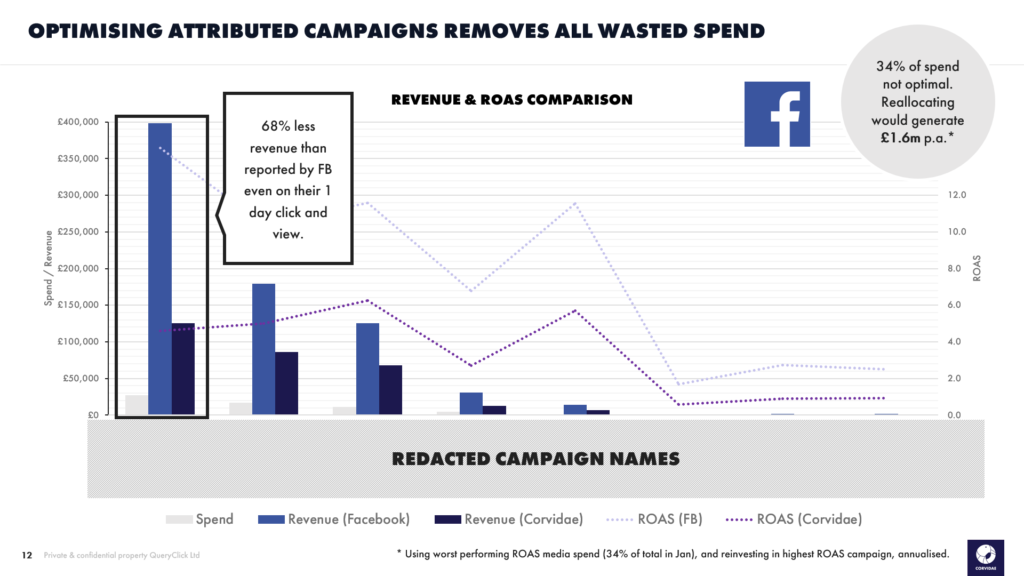
Immediately, a £1.6m increase in revenue simply by reallocating media spend into campaigns doing a better job at influencing customer behaviours in those early pre-consideration phases that are cheaper to place an ad against due – ironically enough – to their poorly reported value using Facebook’s own attribution or your own analytics data.
But of course performance optimisation needs to go deeper again than just campaign level.
With Corvidae, individual adset data is attributable, allowing analysis and improvement of individual creatives within campaigns – the final piece of the puzzle for unified analytics and optimisation of digital marketing activity.
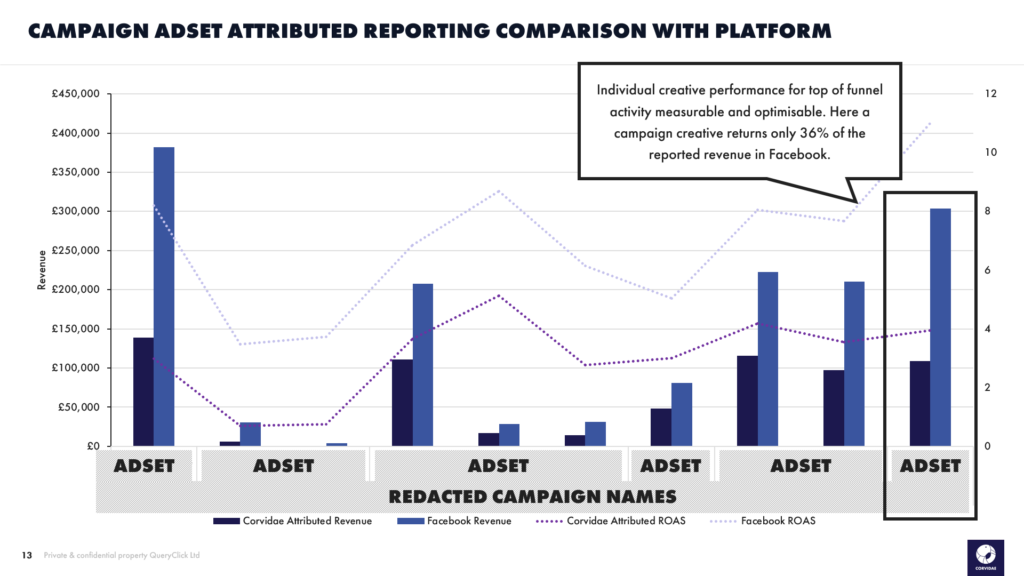
In the example above for a QueryClick client, a creative returned just 36% of the revenue attributed to it in Facebook’s platform, moving it from a top performer to a basket-case of a creative, ripe to be swapped out for a better match to the customer intent of the adgroup audience.
This kind of outcome is ONLY enabled by embracing probabilistic analytics.
The good news is there are other, more powerful benefits from embracing this move from a purely deterministic approach.
Step 2: Include a wider view of your marketing activity
Integrating offline & building a unified analytics view
Once you have embraced de-siloing your digital marketing data with probabilistic analytics, most marketers will want to include all other aspects of marketing behaviour into a single, unified view – the holy grail for marketers.
Fortunately, a problem that is unaccountably complex and expensive to even approach with deterministic approaches becomes radically simpler with an accurate probabilistic, session joining engine like Corvidae.
The main dataset outcome from applying Corvidae’s session rebuilding process is a map of each individual – or entity in the terminology – and their path through all supplied marketing activity, with a scored probabilistic measure of the conversion probability of the session as it passes through each individual data point in its conversion path.
That path has timestamps and latitude and longitude data held against it which – along with the rich associated metadata of the entity – can be used to join data silos of marketing activity where conversion behaviour has increased while the predicted probability of that progression is lower – they have been influenced by something outside the existing dataset.
If that new data overlaps in lat/long and timestamp, then the ad exposure is probable and ‘stitched’ into the entity’s conversion journey.
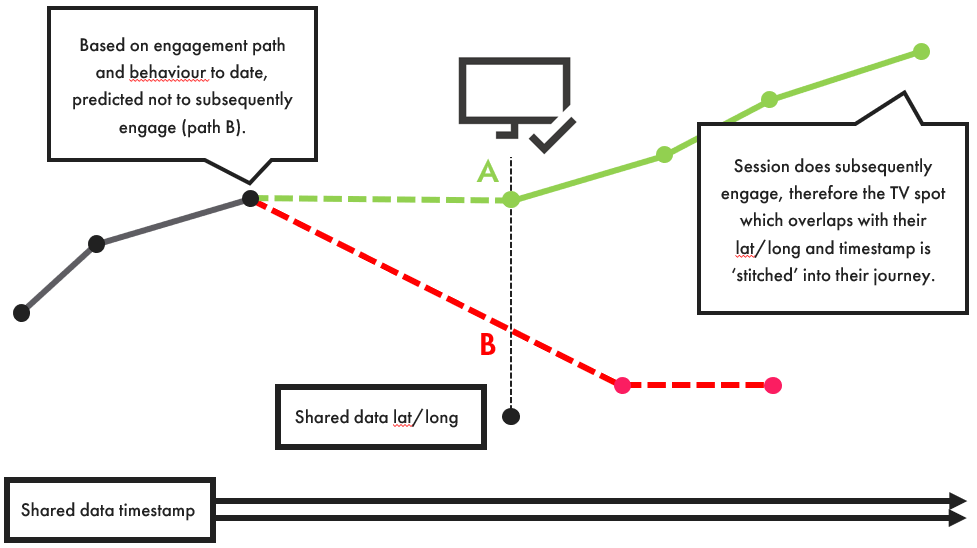
Ultimately, this means that the session stitching technology at the heart of Corvidae, allied with the new deep learning attribution modelling that provides individualised engagement conversion probabilities for each existing and subsequent engagement in an individual entity’s conversion path allows a complete unification of all data sources that offer at least a timestamp and geographic information to a reasonable resolution.
This level of granularity which enables data joining and highly accurate attribution modelling also allows performance marketing optimisation insight into ‘traditional’ media activity, allowing marketers to treat linear TV, OOH or Radio buys in the same way as their digital counterparts.
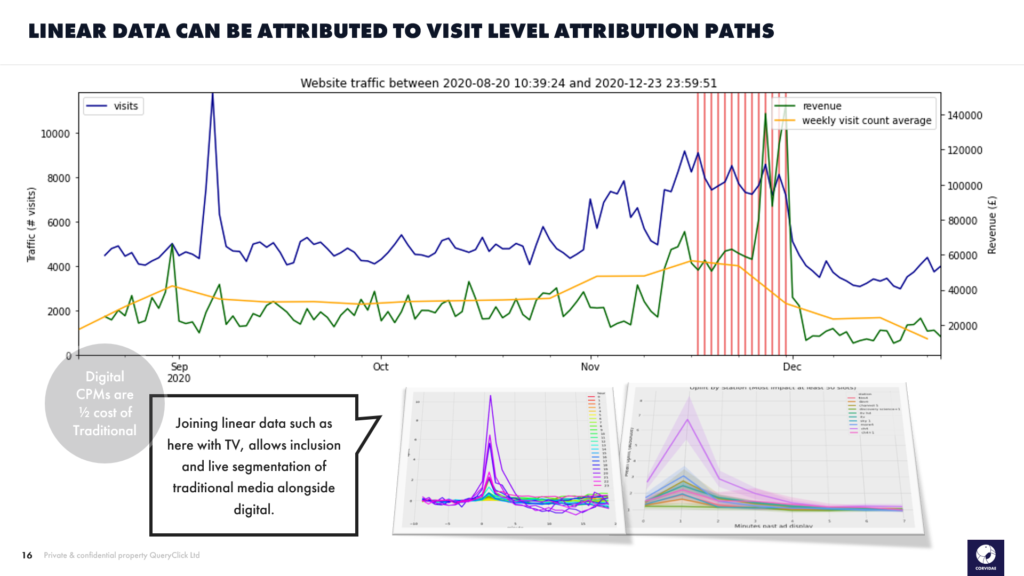
Creating a 1st party data strategy for success
How to achieve accurate attribution without cookies
So, finally, we have a unified, attributed dataset that encapsulates the entirety of our marketing effort in a live access environment (SAAS, dynamic API endpoints), how do we now engage with AdTech to activate most efficiently and find real reach in a modern tech ecosystem?
Well firstly, we need to forget about using our audiences for any form of demographic optimisation.
Studies are now working with enough data to identify that for prospecting – finding new potential customers – we would get a better result with a ‘spray and pray’ campaign, than we would paying for expensive adtech and martech to layer in 2nd party datasets with demographic data.
2nd party, by the way, is when 1st party datasets are shared server-to-server – they are not collected by you, but can be used by you to optimise your marketing spend and develop targeting models if you so choose – and have the money to spend. It essentially takes about 2.5x the cost of your display ad to get good enough quality targeting data to generate an improvement on ‘spray and pray’ in Programmatic today.
Video ad improvements come with a 1.5x premium on the ad cost.
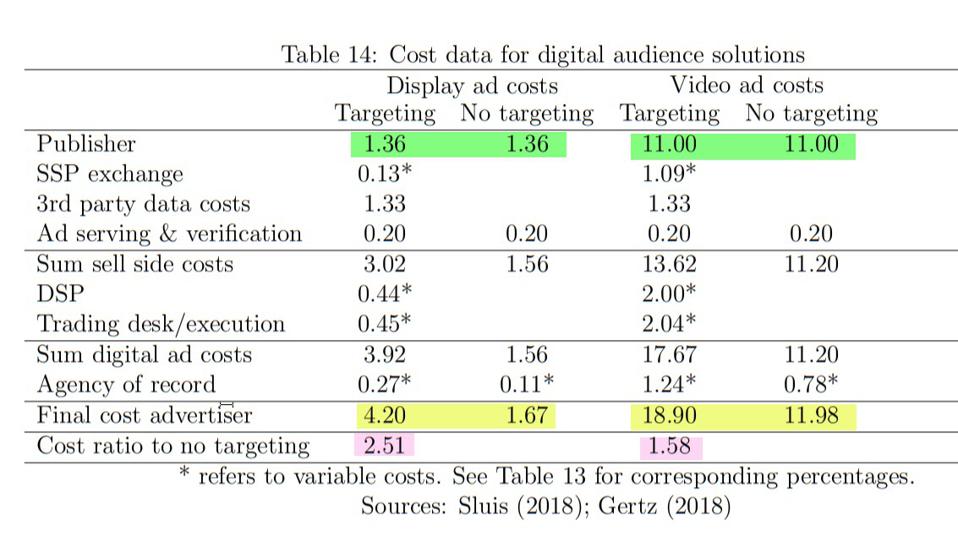
Taken on tandem with the data from adstxt.com on the coverage of ad networks of the total available inventory for programmatic display advertising – 98.55% of which is available through one single network: Google’s Display Network – with the remaining networks essentially offering slices of that overall network, and insight such as that generated by Dr Augustine Fou – that reach ultimately comes from a handful of sites – and the need for complex DSPs which take 15-20% of your programmatic media spend as adtech cost become very questionable.
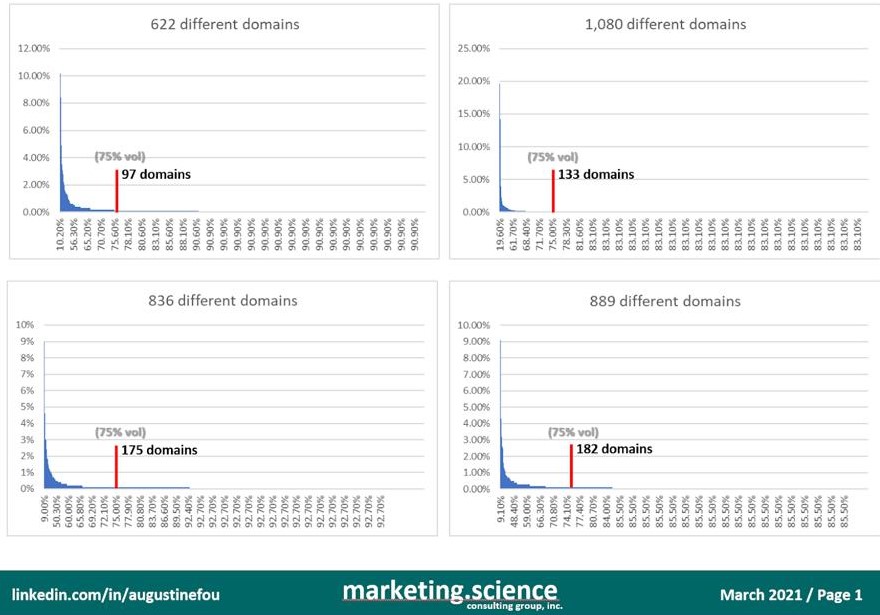
This challenge is compounded by the large slice of programmatic spend that is taken by adtech and martech today as detailed by ISBA in 2020.
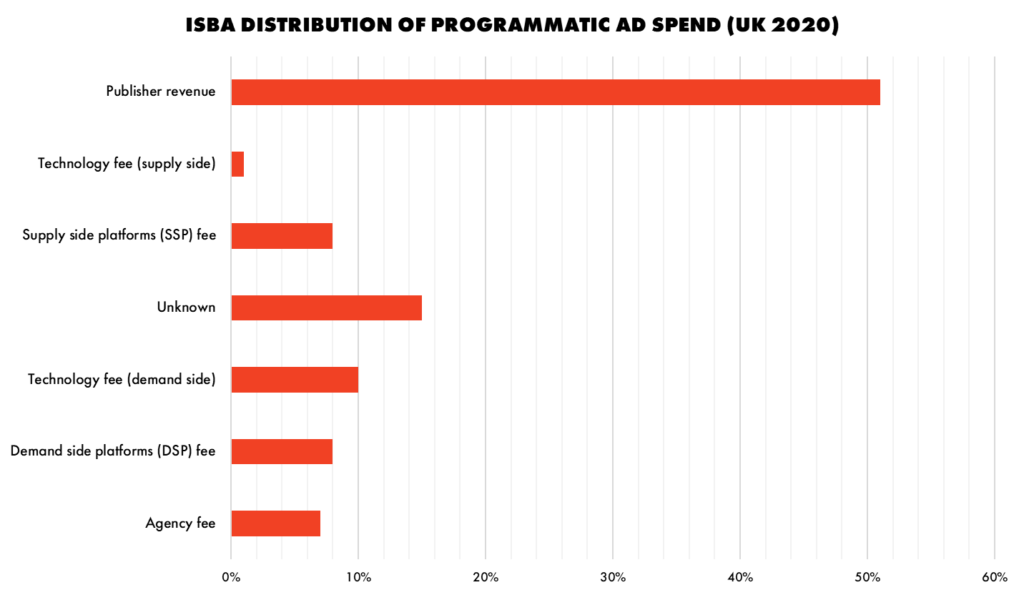
This distribution suggests that demand side cost – including agency management – takes approximately 40% of all programmatic media spend.
We can today return that wasted 40% by simplifying our DSP requirements to creative management and access to the Google Display Network – plus any private marketplace deals we wish to make – by also using our predictive entity graph to generate custom targeting models based on predicted conversion state.
And indeed we have tested that outcome using Corvidae, delivering a side by side improvement over DV360 targeting models of 4X more conversions at ½ the CPA. An outstanding result, and evidence of the power of predictive behaviour-based modelling over old demographic or cookie based targeting.
The other advantage of this approach is it allows elegant and simple joining of 1st party data sets with minimal adtech interference, and uses our own 1st party data to power growth of new customer acquisition into top of funnel activity at significantly lower CPAs than in today’s non-attributed, fragmented and siloed world.
To make that statement easier to visualise, here is a map of the minimal tech architecture needed with Corvidae in the mix as the 1st party data collector that enables 100% reach for the most popular marketing channels today.
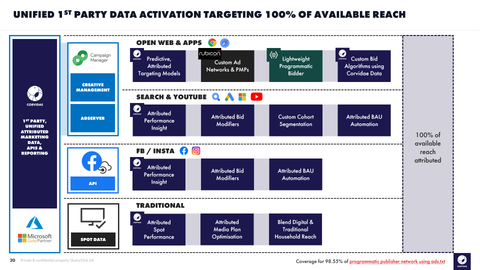
This approach allows the wasted 40% of programmatic spend to be returned to use on additional prospecting activity to drive new customers – targeted using granular attributed ROAS controls to ensure new – incremental -customers are being targeted based on their engagement behaviour and your 1st party targeting data to maximise relevancy and conversion performance.
Using this approach, we also have the ability to integrate offline and unify our household targeting to take advantage of the fact that on a CPM basis it is about half the cost to target a household digitally as it is to use traditional targeting.
In Summary
So today we have an ecosystem that is fit and ready for a 1st party world, and has the added benefit of enabling a unified view of all marketing activity – not just siloed digital snapshots.
Taking advantage of the huge benefits offered to us as marketers from probabilistic analytics is critical in 2021 and beyond to ensure success in a 1st party world.
Corvidae’s deployment’s average ROI is 40:1 for businesses that embrace taking action on the powerful insights delivered by attributed revenue and ROAS.
Discover Corvidae
Own your marketing data & simplify your tech stack.
Have you read?
I have worked in SEO for 12+ years and I’ve seen the landscape shift a dozen times over. But the rollout of generative search engines (GSEs) feels like the biggest...
As you will have likely seen, last week Google released the March 2024 Core Algorithm Update. With it, comes a host of changes aiming to improve the quality of ranking...
After a year of seemingly constant Google core updates and the increasingly widespread usage of AI, the SEO landscape is changing more quickly than ever. With this rapid pace of...